Leveraging Machine Learning for Advanced Materials Discovery and Electromagnetic Applications
Yang Hao
Queen Mary University of London
DIPC Seminar Room
Alexey Nikitin
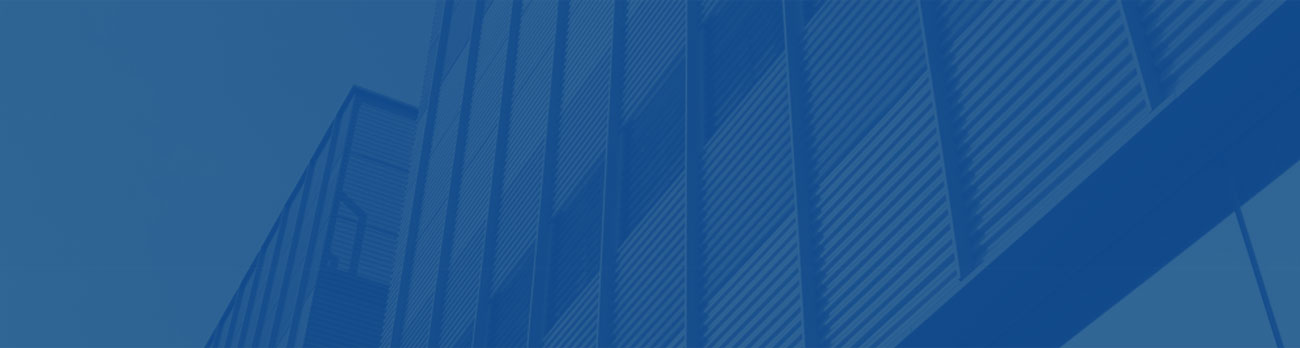
This seminar will explore the transformative role of machine learning (ML) in the fields of materials discovery, antenna design, and electromagnetic applications, emphasising recent advancements made through interdisciplinary research. Machine learning algorithms are proving crucial in accelerating the development of novel materials and optimising complex systems in various applications, including wireless communication and healthcare technologies. Key topics to be covered include: Materials Discovery through ML: Recent studies have demonstrated the power of machine learning in predicting and identifying new materials, such as phase-change materials and perovskite solid solutions. For example, Omidvar et al. (2024) utilized ML-driven automated materials synthesis and characterization to accelerate the discovery of perovskite materials for optoelectronic applications (Omidvar et al., Nature Communications, 2024). Additionally, the self-attention neural network model proposed by Ihalage et al. (2022) provides a representation-domain independent method for discovering new materials using crystal structure information (Ihalage et al., Advanced
Science, 2022). Antenna and Metasurface Optimisation: Several papers
highlight the integration of ML in antenna classification and metasurface optimisation. For instance, Ma et al. (2020) applied Gaussian Mixture Models (GMM) for antenna classification, advancing ML's role in wireless communication systems (Ma et al., IEEE Open Journal of Antennas and Propagation, 2020). Moreover, Chittur et al. (2023) employed active learning techniques to optimise binary-coded metasurfaces, demonstrating how ML can enable efficient designs for wideband meta-atoms (Chittur et al., Sensors, 2023). Healthcare and Electromagnetic Systems: The use of deep learning to optimise large-scale disordered metasurfaces has been explored in several studies, including Ma et al. (2023), who demonstrated the power of deep interactive learning in rapid optimisation for photonic applications (Ma et al., Advanced Photonics Research, 2023). Furthermore, Teoh et al. (2022) applied deep learning for behaviour classification in preclinical brain injury models, showcasing ML's impact on healthcare-related electromagnetic applications (Teoh et al., PLoS One, 2022). Finally, ML has been applied to emotion detection using wireless signals, offering new ways to assess emotional states in non-invasive manners with a deep learning framework for subject-independent emotion detection based on wireless signals (Khan et al., PLoS One, 2021). This work paves the way for more sophisticated human-machine interaction technologies, particularly in therapeutic and diagnostic contexts.